How Can AI Help Transform Radiology?

At UW Health in Wisconsin, radiologists have started to embrace the use of artificial intelligence throughout their workflows.
Team members have adopted AI image reconstruction algorithms for the MRI, CT scan and PET scan machines, enabling faster captures and higher-quality images.
AI algorithms are also in use to analyze medical imaging and help diagnose patients. For example, as part of the emergency department triage process, computer-assisted detection tools can flag potential abnormalities in scans and then prioritize them for review, says Dr. Scott Reeder, radiology department chair at the UW School of Medicine and Public Health.
“We see cases where an X-ray is acquired, and a minute or two later, it’s flagged and pops up on the workstation,” says Reeder, who is also a radiologist at UW Health, the academic medical center affiliated with the university. “So, I read that case next, and sure enough, if there is an abnormality, I call the emergency physician and say, ‘This patient has this urgent finding,’ and they can treat the patient immediately.”
Healthcare is grappling with workforce shortages and clinician burnout in multiple disciplines, including radiology. As AI and machine learning capabilities continue to improve, providers hope that AI-assisted radiology tools will help the experts better parse images for more precise clinical decision-making and alleviate administrative burdens.
Click the banner below to read the new CDW Artificial Intelligence Research Report.
Academic medical researchers and tech companies are combining their efforts to develop these new tools. Initial solutions are showing positive results for improving patient care and streamlining operations, radiologists say.
To seamlessly adopt AI in radiology, healthcare organizations can take advantage of their existing technology infrastructure, including medical imaging storage systems, but they must rethink parts of their enterprise, says Mutaz Shegewi, senior research director at IDC Health Insights.
“They need workflow integration. They need computing power, and they’re going to need governance and security,” Shegewi says.
Faster Scans and Improved Emergency Room CareAs UW radiology researchers explore the adoption of AI in their field, they need to keep in mind that only AI technology approved by the U.S. Food and Drug Administration can make it to their health system, Reeder says.
For example, UW Health has incorporated FDA-approved advanced image reconstruction in its scanning machines, which produces sharper images with reduced image noise and artifacts and cuts scan time by 30% to 50%, which can reduce a patient’s exposure to radiation, he adds.
“Patients like it. We like it too, because it means we can schedule shorter exam slots,” Reeder says. “It improves throughput and workflow. It’s a game changer.”
To power AI tools in radiology, UW Health relies on in-house servers, the cloud, general purpose computers and the imaging machines themselves, just to name a few, says John Garrett, director of imaging informatics in UW’s radiology department.
For instance, while some AI models run on standard computers, AI-powered CT and MRI brain perfusion software, which analyzes brain scans and produces color-coded images of how blood flows in the brain, runs on an on-premises server, he adds.
EXPLORE: Unlock data flow with high-performance storage for medical imaging and analytics.
Exams that need more computing power run on GPUs in the cloud, such as CT scans that get evaluated by 10 to 12 AI triage tools, Garrett says. Depending on the specific algorithm, Amazon Web Services and Microsoft Azure perform real-time data processing, while some tools use Google Cloud Platform.
In emergency department triage situations, data is sent to the cloud, where it is processed by multiple AI algorithms. The results are sent back to UW Health’s picture archiving and communication system. A desktop widget on radiologists’ PACS workstations can alert them of high-priority findings, Garrett says.
UW Health radiologists also use AI-powered voice recognition software Nuance PowerScribe on their Dell computers to help with report writing. Reeder has been a longtime user of the solution, which he notes has continuously improved.
“It allows us to generate reports efficiently, accurately and in a standardized way,” he says. “While it’s not perfect, it is accurate. You can say fancy medical words and it gets it right.”
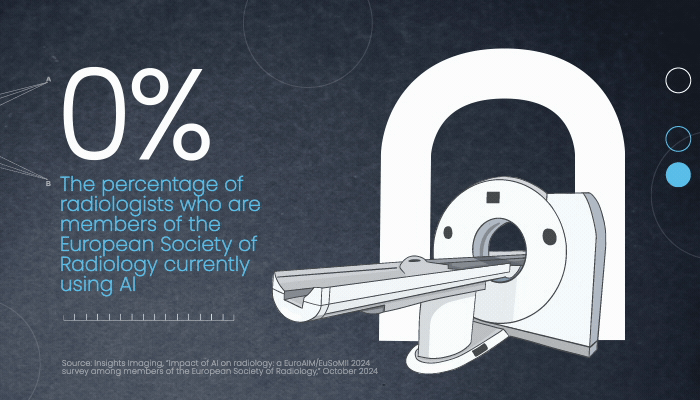
Boston-based Massachusetts General Brigham has adopted various radiology AI tools in its clinical practice. These include image processing tools used to improve image quality and perform “image quantification,” which can assist radiologists in reading medical images, says Dr. Bernardo Bizzo, senior director of Mass General Brigham’s AI business.
The health system has deployed AI-powered, computer-aided triage tools that help prioritize and notify radiologists of urgent findings, he says. It has also tested dozens of AI models that analyze medical images, and so far has adopted about a dozen of them, Bizzo says.
Mass General Brigham runs these AI tools in on-premises data centers and the cloud. It has adopted some AI models within Microsoft’s Nuance Precision Imaging Network (built on Azure), for instance, and has deployed models that detect pulmonary nodules in CT scans and analyze liver MRI scans.
RELATED: Transform MRIs with modern scanners and virtual programs.
These AI tools help radiologists with interpreting scans, but current benefits are limited because each model is generally designed to detect one or a few findings. Radiologists must take a holistic view and analyze the image for a comprehensive report, Bizzo adds.
“Each FDA-cleared AI tool detects only a few imaging findings. It’s helping a bit on specific use cases, but it’s not moving the needle in the way we all hope that AI will, because the work of a radiologist goes beyond just detecting a handful of findings,” he says.
However, radiologists say they are confident that the nascent AI tools can mature. For example, Mass General Brigham works with companies worldwide to test their emerging AI models and tools as they seek FDA approval and clinical use in the U.S. That includes testing generative AI and comprehensive AI tools. These new tools may combine multiple algorithms into a single package to perform more in-depth diagnoses, Bizzo says.
“In my opinion, AI tools for detection and diagnoses can help radiologists the most, especially when drafting reports in the near future,” he says. “There is a lot of promise for AI to enhance diagnostic accuracy and efficiency.”
AI Tool Measures Kidney Disease Treatment EffectivenessAbout a year ago, Weill Cornell Medicine’s radiology department built an advanced AI cluster – powered by Dell servers running on NVIDIA GPUs – that enables researchers to develop and train AI models for radiology, then use and test them in a clinical setting.
For example, physicians at New York-Presbyterian Hospital/Weill Cornell Medical Center (the medical school’s research and teaching hospital) are currently testing an AI model to take precise measurements of patients’ kidneys to determine disease progression and treatment effectiveness, says Dr. Mert Sabuncu, vice chair of AI and engineering research at Weill Cornell Medicine’s radiology department and professor of electrical and computer engineering at Cornell Tech.
More specifically, the AI model was designed to analyze clinical scans of patients with polycystic kidney disease, a genetic disorder in which cysts form and enlarge in the kidneys, which can lead to kidney damage and failure.
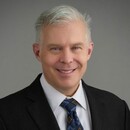
Dr. Scott Reeder Radiology Department Chair, UW School of Medicine and Public Health
Patients with advanced PKD typically get an MRI once a year.
“Standard radiology reports have a lot of impressionistic statements,” Sabuncu says. “Radiologists describe what they see without a lot of quantitative measurements, which means it can take several years to figure out if a drug like Tolvaptan is working.”
A few years ago, a research team at Weill Cornell Medicine developed an AI model that can produce highly precise numerical measurements of abdominal anatomy. Today, this tool is used to inform clinical decision-making and to better assess whether a drug is effective for patients, he says.
AI can make mistakes, so as part of the workflow, a technologist or radiologist reviews the model’s results, makes any corrections necessary, and then forwards it to another radiologist for final review. They can also reject the results if the AI model’s measurements are wrong.
“We have a human in the loop to ensure accurate results,” Sabuncu says.
Weill Cornell Medicine hopes to gain FDA approval for the AI model, but for now, it can use it on patients as an academic research hospital. “We have the luxury of being able to use cutting-edge research tools,” he says.
He believes that such tools are poised to revolutionize radiology. “We are on the cusp of going through a big transformation over the next five years. There’s going to be a huge influx of AI tools that will enhance radiology and transform medicine,” Sabuncu says.
healthtechmagazine